A case study of patients with hypercholesterolaemia in North West London
Prediction of cardiovascular adverse events and lipid lowering treatment optimisation
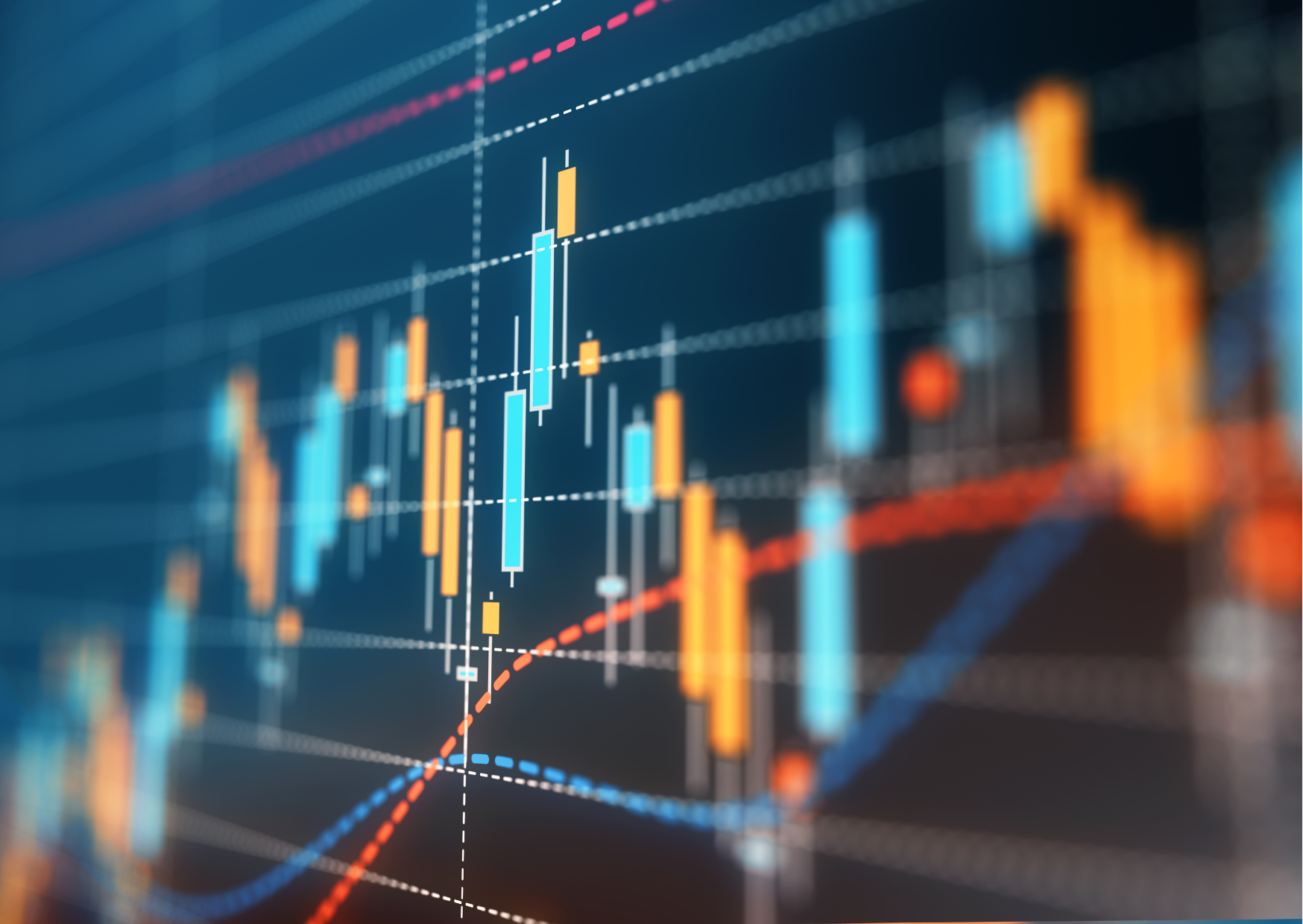
Background
4The NHS Long Term Plan includes a major ambition to prevent 150,000 strokes and heart attacks over the next 10 years by treatment for high-risk conditions such as high cholesterol, hypertension (high blood pressure) and atrial fibrillation (AF). These common conditions cause cardiovascular disease (CVD), which can cause heart attacks, strokes, and cases of dementia.
The NHS Long Term Plan also focuses on Familial hypercholesterolaemia (FH) which affects at least 150,000 people in England, but only 7% of cases were diagnosed as of 2019/20. The ambition is to increase levels of diagnosis to 25% by 2023-24 through improving patient identification and access to genetic testing.
To support this aim, Daiichi Sankyo wish to provide more detailed real-world data insights and resources to the NHS to build a robust evidence-base for change, specifically in the area of hypercholesterolaemia (HC) pathways.
By identifying risk factors/patient cohorts most ‘at risk’ and most impactable to interventions, clinicians and commissioners will be able to better target resources to reduce cholesterol levels across the population in North West London (NWL), supporting the aim of saving lives by reducing heart attacks and strokes.
The Discover dataset is an important advance in the provision of data banks in the UK as it contains linked, coded data from primary and secondary care, and is therefore uniquely able to provide a whole system view of the patient journey throughout their medical care.
Aim of the study
- Analyse demographics and clinical characteristics of HC and FH patients who are currently receiving treatment in-line with National Guidance for Lipid Management for Primary and Secondary Prevention of CVD, and the population who would benefit from treatment optimisation.
- Use machine learning to find factors which are associated with cardiovascular risk as well as lipid lowering treatment optimisation in these patients, i.e. identify the treatment gap for those that are sub-optimally managed and ‘at risk’ and could benefit from intervention to reduce risk of future CVD events.
Methodology
Two cohorts were defined and described in the study using the Discover dataset: identified cohort (people with (familial) hypercholesterinaemia) and at-risk cohort (people with conditions predisposing to cardiovascular disease – hypertension, diabetes, chronic kidney disease, alcoholism, atrial fibrillation and some other conditions).
The study included four tasks:
- Predicting cardiovascular adverse events in the identified cohort using DataRobot AI Cloud.
- Predicting lipid lowering treatment optimisation in the identified cohort using DataRobot AI Cloud.
- Descriptive analysis of cardiovascular adverse events in the at-risk cohort.
- Predicting cardiovascular adverse events in identified cohort with data about statin optimisation and impact on LDL level using logistic regression.
Outcomes
Exploratory analysis of the identified cohort showed that only 40% of patients with hypercholesterinaemia receive statins or other lipid lowering drugs. High-intensity statins were prescribed to ~10% of patients and this dropped to ~5% for the initially prescribed drug at 15 months. The study found that frailty is an important factor for predicting cardiovascular events as well as lipid lowering optimisation. Being classed as severely frail predicted a 24% chance of a change in lipid lowering therapy and 7% chance of a cardiovascular event within 2 years.
The project highlighted the value of real-world evidence in understanding the factors contributing to cardiovascular risk and may help to develop the Hub expertise in supporting studying of hypercholesterolaemia. The study is also planned be published in a peer-reviewed article and could lead to improving policies in management of hypercholesterolaemia patients.