News
Using linked data to test predicative algorithms
Sara Sekelj, Data Analyst, tells us how ICHP is using its unrivalled access to linked depersonalised health data to test predictive algorithms developed by industry leaders.
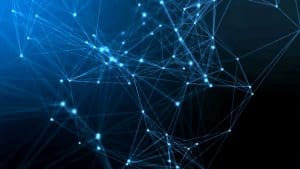
At ICHP we have access to one of the largest depersonalised linked datasets in Europe through Discover-NOW Health Data Research Hub for Real World Evidence as one of seven Health Data Research UK’s Hubs. In a safe, secure and trusted environment it enables clinicians, researchers scientists, supported by the people of NWL, to accelerate the development of new treatments, devices and apps to more effectively manage, and even prevent health conditions through research.
At ICHP we aspire to lead the way in how data can be used to transform health and care in the future. We do this through closely collaborating with our partners, members and sector leaders to use the data for healthcare research. One such collaboration this year has seen us working with Bristol Myers Squibb on behalf of the Bristol Myers Squibb-Pfizer Alliance to test its algorithm designed to predict who may be at risk of developing atrial fibrillation (AF), which is on the rise globally with a significant amount of health resources invested into detecting and managing it.
The AF predictive algorithm was developed in Clinical Practice Research Datalink (CPRD) a nationally representative primary care data set, with the aim of more effectively screening the population to see who has a risk of AF and to find more patients with AF.
We were approached to test the algorithm in a real-life scenario using the rich depersonalised dataset of 2.5million patients. This dataset is compiled from 400 provider organisations including ~360 GP practices, two mental health trusts, two community trusts, social care and all the acute providers within the North West London region.
We applied the algorithm to the dataset to find people’s risk scores for developing AF by looking at their GP records for height, weight, age and other medical issues such as hypertension or heart failure. In real life there are different methods of measuring someone’s risk of developing AF, so this was an ideal opportunity to see how the algorithm would work in practise. We wanted to see if the algorithm was generalisable, if it produced any useful outputs and if there were any impacts on its performance when used in a different data set.
The algorithm was successfully ran obtaining insights for Bristol Myers Squibb on behalf of the Bristol Myers Squibb-Pfizer Alliance. Our team is now undertaking an economic evaluation of the algorithm as phase two of the project.
We were excited to work on this cutting-edge collaboration building our knowledge and expertise in running algorithms through this depersonalised linked dataset and applying it in practice.
We now know it’s possible to do this crucial testing with our advanced system and we are keen to continue innovative collaborations of this sort in the future.
Download news story
DownloadRelated posts
Our services
Transforming the delivery of healthcare through data
Our team can work with throughout the life spam of your project
Our services