Case study
Predicting Complications in Diabetes Patients
Through the Risk Algorithms for Decision Support and Adverse Outcomes Reduction (RADAR) project we are aiming to develop and test data-driven apps and dashboards, that deliver better information visualisation and decision support directly to front-line clinicians and patients.
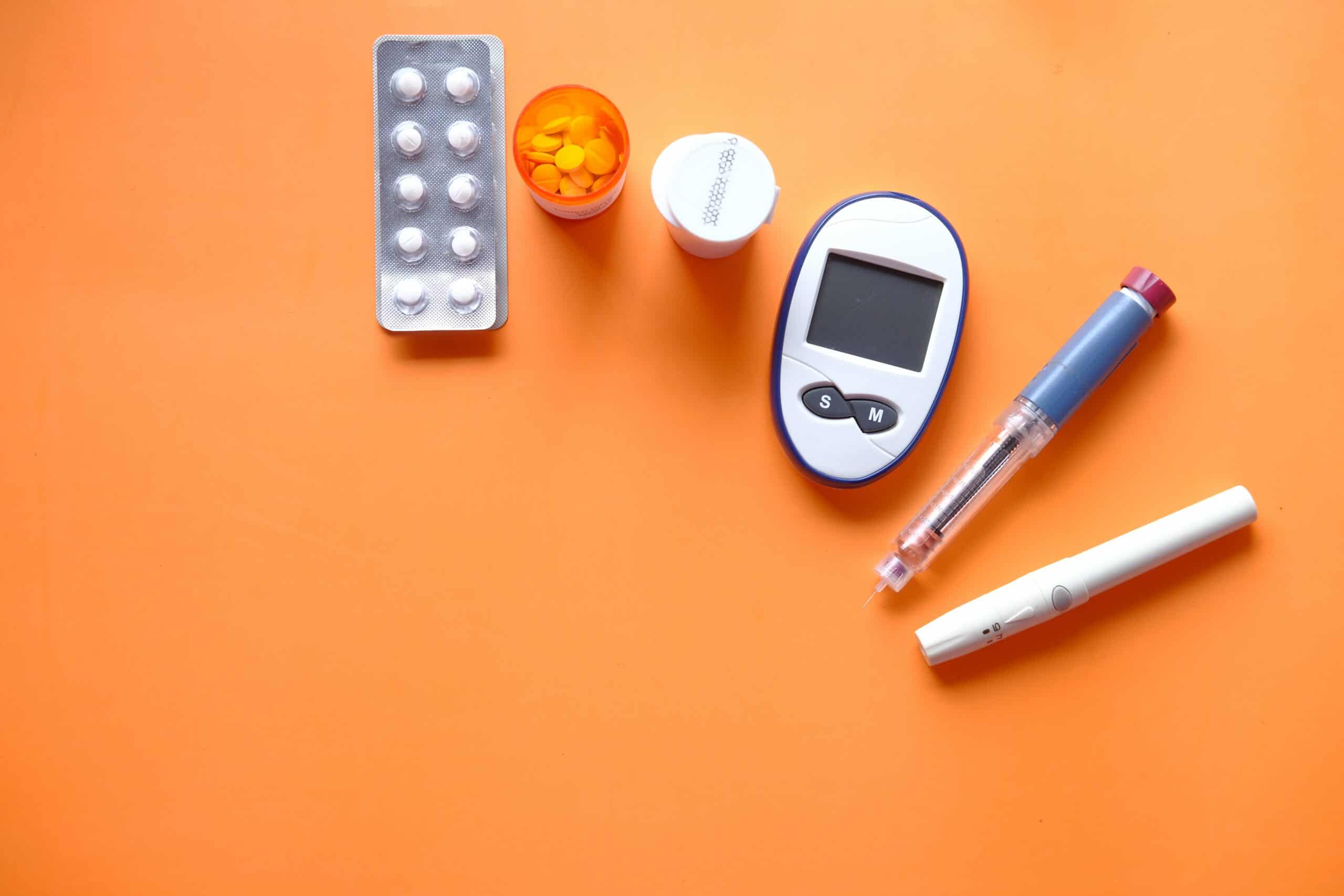
Background
With Type 2 Diabetes Mellitus (T2DM) increasing a patient’s mortality risk from COVID-19 by as much as 25%, it is no surprise to find that many sufferers are choosing to avoid clinical settings during the pandemic. However, this shielding has resulted in a fall in primary care appointments for T2DM patients, which in turn makes it harder for clinicians to predict the likelihood of complications arising and provide interventions before they do.
Through the Risk Algorithms for Decision Support and Adverse Outcomes Reduction (RADAR) project we are aiming to develop and test data-driven apps and dashboards, that deliver better information visualisation and decision support directly to front-line clinicians and patients.
As part of this we will be looking at existing Artificial Intelligence (AI) models, which have been developed and successfully used in Scotland, and using our Discover depersonalised longitudinally linked dataset to test these models in North West London.
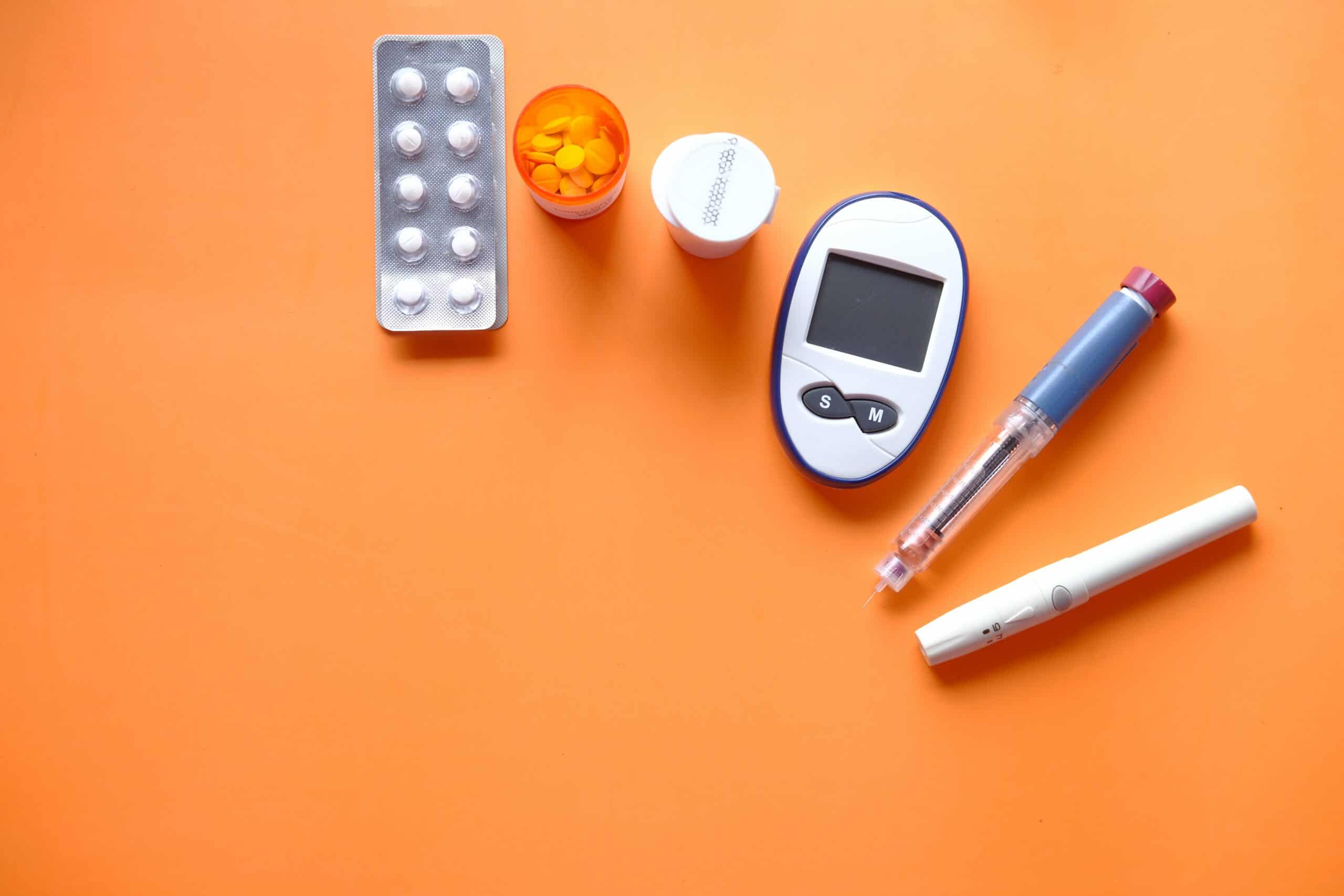
Study aims
The need for predicting complications
Diabetes is a growing global health challenge with massive attributable costs, mainly due to long term complications that could be avoided. Data driven solutions may be able to deliver scalable low-cost management and/or self-management support to clinicians and patients to improve the outcomes, safety, and quality of life for patients and reducing the health care burden.
As the prevalence of T2DM, commonly known as Type 2 Diabetes, grows the burden on healthcare is increasing in the form of additional consultations, hospital outpatient visits, death, and disability.
T2DM represents a large part of the disease and cost burden of healthcare in North West London (NWL) to the value of £600m annually. Patients with T2DM constitute over 40% of all NWL admissions, with complications of diabetes representing a significant factor in the morbidity and cost associated with this chronic disease.
Mortality risk from COVID-19 is approximately 25% higher in patients with T2DM and shielding has resulted in reduced primary care appointments for patients with T2DM. Predicting the risk of people developing complications from diabetes hopes to strengthen population health management by providing better-tailored services and proactive interventions.
RADAR
Project aims
This project aims to develop and test data-driven end-user tools, such as apps or dashboards which can be viewed on a computer, that deliver better information visualisation and decision support directly to front-line clinicians and patients. Specifically, it will evaluate the use of risk prediction, powered by a machine learning algorithm, as a catalyst for change around early intervention, both in terms of medical management and patient self-management.
The research has two aims:
- Develop methodology to rapidly revalidate three artificial intelligence models developed in Scotland in NWL datasets to help predict risk of complications for patients with T2DM.
- Assess how these models can best drive improved clinical outcomes specifically within the NWL Ecosystem
Partners
Who is doing the research?
We have collaborated with multiple partners on this project, through our Discover-NOW Hub, and led by North West London Health and Care Partnership (NWL HCP). The other project partners are: The Institute of Global Health Innovation (IGHI), Imperial College London, Imperial College Healthcare Trust, MyWay Digital Health (MWDH) and AstraZeneca (AZ).
Methodology
How the research is being carried out
The project is being carried out by:
Making use of existing diabetes predictive AI models developed in Scotland (developed by MyWay Digital Health).
Applying these to the North West London (NWL) population, by revalidating in NWL’s longitudinally linked dataset, Discover.
Considering, developing, and iterating prototypes for end-user interfaces that use risk prediction to drive clinician and patient behaviour change impacting on clinical decision making and patient self-management behaviour.
“Needs assessing” end-user requirements, UX and UI requirements and wider facilitators and barriers to success adoption
Testing and evaluating prototypes with clinicians and individuals with Type 2 Diabetes.
Patient and Public Involvement
Project feedback
The project team delivered a series of workshops in January and February with patients living with Type 2 Diabetes in order to gather their feedback on risk prediction tools designed to reduce complications, as well as to gather insight on how conversations about risk are best managed. Valuable feedback was gathered, consolidated and fed into the iteration and will be part of the further development of the risk prediction tools.
Outcomes
What is next?
This project resulted in significantly improved prediction accuracy of artificial intelligence models to provide calculated risk information, which enables better-informed decisions around treatment and self-management for diabetes patients. These decisions can support improved quality of life, reduce morbidity and the healthcare burden through identification and treatment of high-risk individuals.
Patient feedback also showed a real appetite for the use of these type of tools and the desire for patients to engage more with their own data. Personalised risk profiling can be a driver for patient behaviour change impacting on short-term markers such as weight, glucose control, medication adherence, foot care and ultimately reducing complications risk factors.
Using diabetes as an exemplar, this project will provide the opportunity to use real-world data for the improvement of outcomes in patients with other long-term conditions.