Case study
Atrial fibrillation (AF) risk prediction algorithm
Atrial fibrillation (AF) risk prediction algorithms can aid practitioners in targeted diagnosis of AF. We investigated whether implementing such an algorithm could improve AF detection and reduce costs compared with opportunistic screening during a primary care visit (standard care).
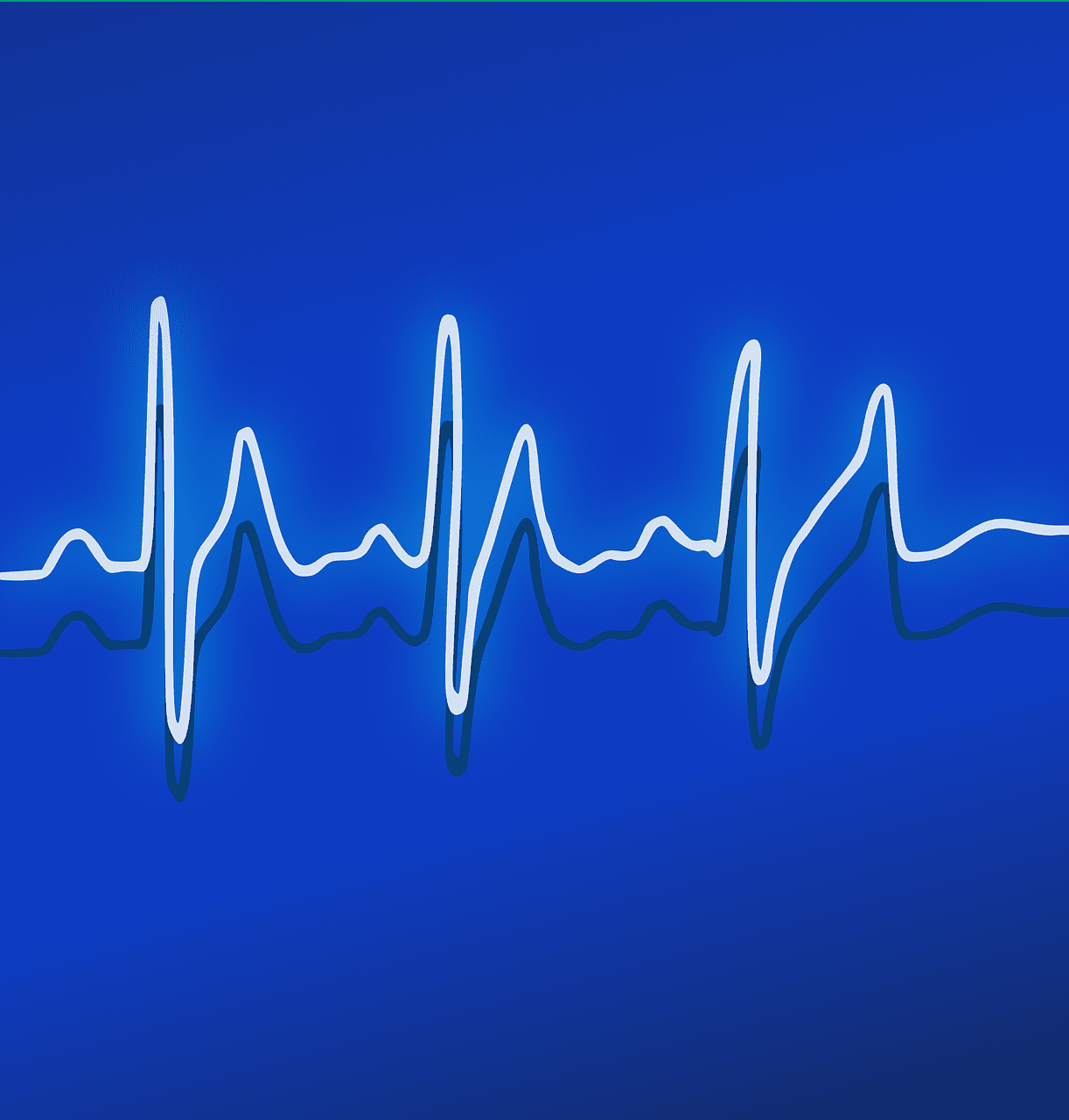
Overview
Atrial fibrillation (AF) risk prediction algorithms can aid practitioners in targeted diagnosis of AF. We investigated whether implementing such an algorithm could improve AF detection and reduce costs compared with opportunistic screening during a primary care visit (standard care).
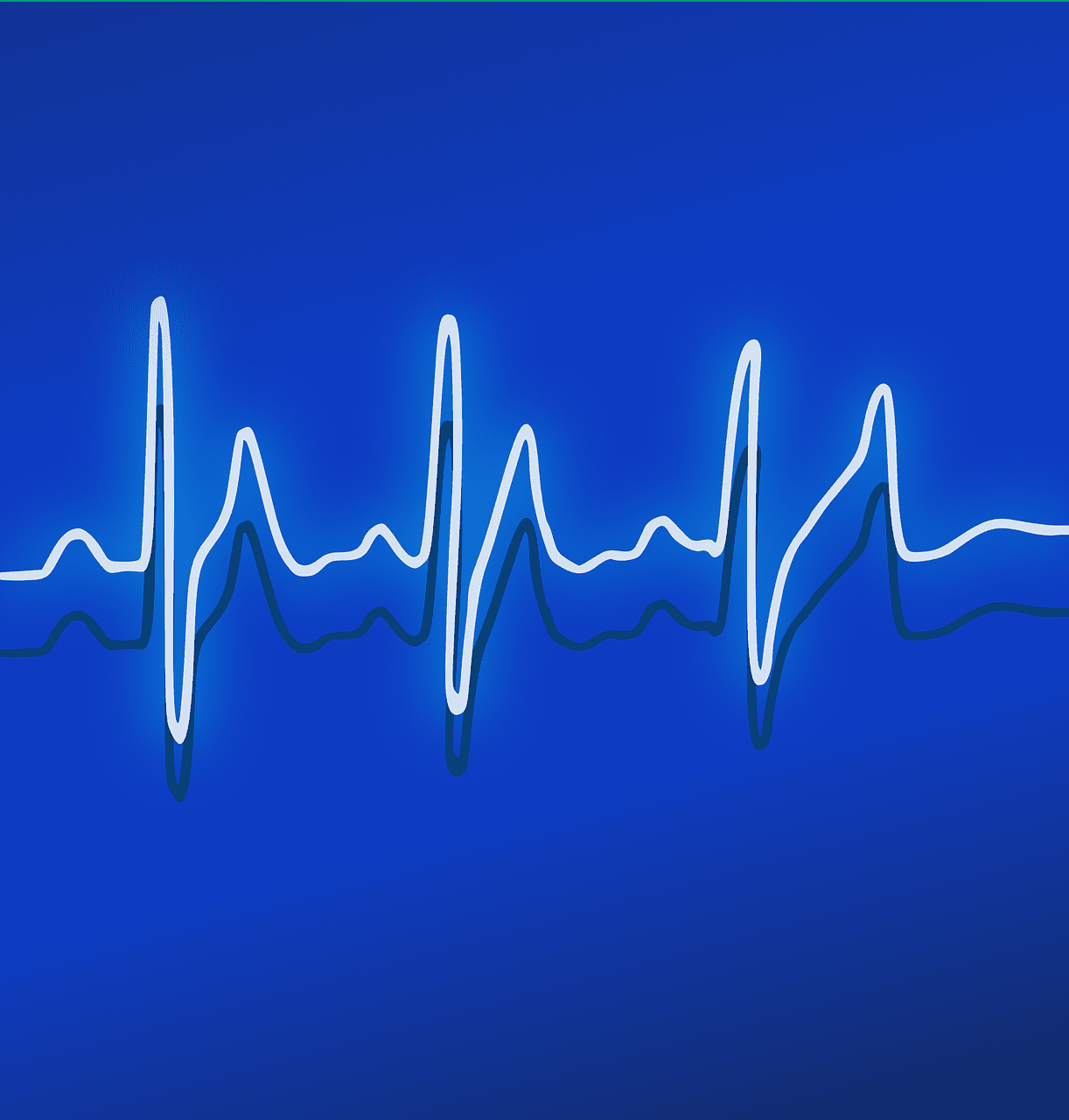
Working in partnership
At ICHP we aspire to lead the way in how data can be used to transform health and care in the future. We do this through closely collaborating with our partners, members and sector leaders to use the data for healthcare research. One such collaboration this year has seen us working with Bristol Myers Squibb on behalf of the Bristol Myers Squibb-Pfizer Alliance to test its algorithm designed to predict who may be at risk of developing atrial fibrillation (AF).
The AF predictive algorithm was developed in Clinical Practice Research Datalink (CPRD) a nationally representative primary care data set, with the aim of more effectively screening the population to see who has a risk of AF and to find more patients with AF.
Method
Using an budget impact analysis we considered patients registered in England aged 65 years or older in 2018/19. The AF risk prediction algorithm searched for those with complete data for weight, height, body-mass index, and systolic and diastolic blood pressure recorded within a one-year period, which were used to calculated AF risk scores.
We assessed three screening scenarios: 1) standard care of routine clinical diagnosis and opportunistic screening; 2) standard care replaced by use of the algorithm to flag patients who should be invited to attend the practice for assessment; and 3) a combination of scenarios 1 and 2. Budget impact considered direct NHS costs without and with personal social service (PSS) community care costs over a 3-year period.
The impact
Over 3 years, scenario 1 would identify 79,410 new AF cases, which would reduce the detection gap by 22%. With scenario 2, 70,916 cases would be identified, reducing the gap by 19%, and with scenario 3, 99,267 cases would be identified, closing the detection gap by 27%. This translates into 2,639 strokes being prevented in scenario 1, 2,357 in scenario 2, and 3,299 in scenario 3. The NHS budgetary impact of scenario 1 would be £45,320,941, compared with £46,337,471 (difference 2.2%) in scenario 3. In contrast, scenario 2 would cost only £3,630,464 (‒92.0%). However, when considering PSS, scenario 1 would save £48,821,570, whereas scenario 2 could increase savings by £80,441,386 (64.8%) and scenario 3 by £71,345,158 (46.1%).
Conclusions
Implementing an AF risk prediction algorithm alongside routine care would close the detection gap, prevent strokes, while doing so at a reduced cost.
Next steps
We were excited to work on this cutting-edge collaboration building our knowledge and expertise in running algorithms through this depersonalised linked dataset and applying it in practice.
We now know it’s possible to do this crucial testing with our advanced system and we are keen to continue similar innovative collaborations in the future.